The rise of artificial intelligence has led to the creation of highly realistic AI-generated images. While these images have applications in design, entertainment, and marketing, they also pose risks. AI-generated visuals can be used for deepfakes, misinformation, identity fraud, and digital manipulation. As AI-generated content becomes more sophisticated, distinguishing real from synthetic images is crucial for maintaining trust in digital spaces.
AI-generated image detectors are tools designed to analyze, identify, and flag synthetic content. They use deep learning, pattern recognition, and forensic analysis to detect images created by AI. These detectors are essential for security, media integrity, and fraud prevention. This article explores how AI-generated image detectors work and why they are important in today’s digital landscape.
The Technology Behind AI-Generated Image Detectors
Deep Learning and Neural Networks
AI-generated image detectors rely on deep learning models trained on large datasets of real and synthetic images. These models use neural networks to identify patterns unique to AI-generated content. Neural networks mimic the human brain’s ability to process visual information, enabling them to detect inconsistencies that may not be visible to the naked eye.
By analyzing millions of images, these models learn to recognize the common traits of AI-generated visuals. They examine pixel-level patterns, irregular textures, and unnatural symmetry.
Tools like AI or Not leverage these deep learning techniques to determine whether an image is AI-generated or real. As AI-generated images evolve, deep learning models continuously adapt by learning from new datasets to improve detection accuracy.
Pattern Recognition and Image Analysis
AI-generated images often exhibit certain anomalies that real images do not. These include:
- Unnatural Textures: AI models sometimes create overly smooth skin, repetitive patterns, or inconsistent lighting.
- Asymmetry in Facial Features: Human faces are naturally asymmetrical, but AI-generated images often struggle to replicate subtle differences in facial structures.
- Blurred Backgrounds and Mismatched Details: AI-generated images may have elements that blend unnaturally, such as distorted fingers, inconsistent lighting, or unrealistic hair strands.
Pattern recognition algorithms scan images for these anomalies. They compare different areas of an image to detect inconsistencies, ensuring that AI-generated content is identified accurately.
Metadata and Compression Analysis
Beyond visual inspection, AI-generated image detectors also analyze metadata. Real photographs contain metadata such as camera settings, location data, and timestamps.
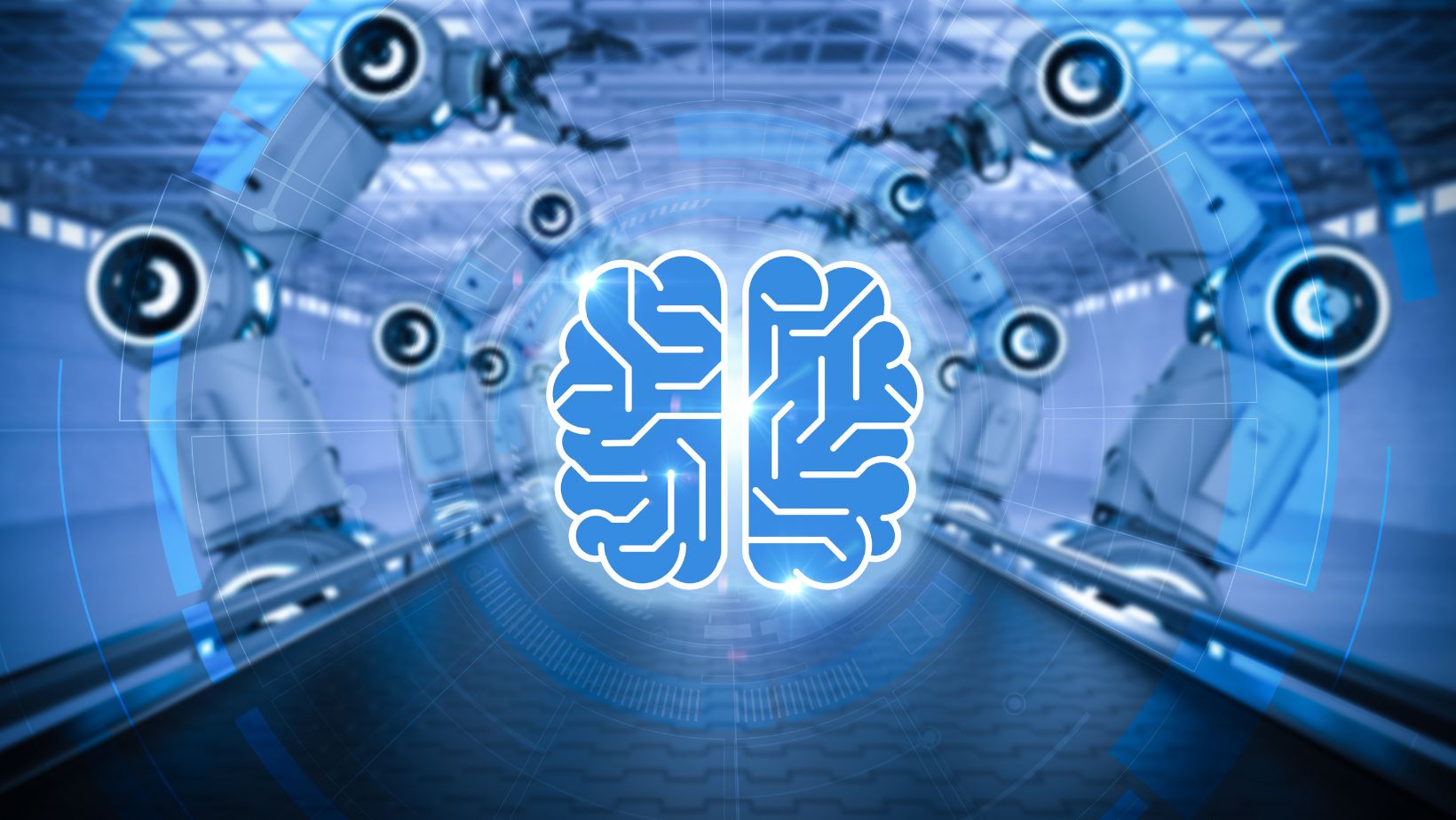
AI-generated images often lack this information or have metadata that suggests they were created using an AI model.
Compression artifacts also play a role in detection. AI-generated images sometimes display unnatural pixel compression, particularly in areas with fine details. Advanced image detectors analyze these compression patterns to determine if an image is synthetic.
AI Forensic Analysis
Some detectors use forensic techniques to examine the source and history of an image. AI forensic analysis includes:
- Error Level Analysis (ELA): Detects inconsistencies in image compression to highlight manipulated areas.
- Fourier Transform Analysis: Analyzes frequency patterns to detect AI-generated textures.
- Noise Analysis: Examines how noise is distributed across an image, as AI-generated images often have different noise patterns compared to real photos.
These forensic methods help improve detection accuracy, especially in professional settings such as journalism and cybersecurity.
Why AI-Generated Image Detectors Matter
Preventing Fraud and Identity Theft
AI-generated images can be used to create fake identities, bypass security systems, and commit fraud. Fraudsters use synthetic images for:
- Fake Social Media Profiles: AI-generated faces are often used to create fake accounts for scams, misinformation, and impersonation.
- Financial Fraud: Fraudsters create synthetic identities with AI-generated ID photos to bypass banking security measures.
- Phishing Attacks: AI-generated profile pictures are used to make phishing emails and fake websites appear legitimate.
AI image detectors help prevent these fraudulent activities by verifying whether an image is authentic or AI-generated. Businesses and financial institutions rely on these tools to ensure secure identity verification.
Combating Deepfake Misinformation
Deepfake images and videos are used to spread false information. These AI-generated visuals can manipulate public opinion, distort reality, and damage reputations. Deepfake misinformation is a growing concern in:
- Politics: AI-generated images of politicians or public figures can be used to create false narratives.
- News and Journalism: Fake images can mislead audiences and undermine trust in media organizations.
- Social Media: Manipulated visuals spread rapidly, influencing public perception and decision-making.
AI-generated image detectors help combat deepfake misinformation by analyzing content before it spreads. Social media platforms and news agencies use these tools to verify images and ensure the authenticity of visual content.
Enhancing Digital Trust and Content Verification
In a world where AI-generated content is becoming more common, maintaining trust in digital interactions is essential. Businesses, media organizations, and security agencies use AI-generated image detectors to verify content and prevent manipulation. These tools ensure that digital spaces remain authentic, protecting users from deception.
The next section will explore the challenges of AI-generated image detection, future advancements, and how different industries benefit from these tools.
Challenges in AI-Generated Image Detection
Continuous Advancements in AI Image Generation
As AI technology improves, detecting synthetic images becomes more difficult. AI models, such as Generative Adversarial Networks (GANs), continue to evolve, making their output more realistic.
Modern AI-generated images can now include imperfections, realistic lighting, and detailed textures, reducing the effectiveness of traditional detection methods.
Detection tools must constantly update their models to stay ahead of new AI-generated image techniques. If detection algorithms fail to evolve, they may become ineffective in identifying synthetic content. Researchers and AI developers work together to improve detection accuracy, but the challenge remains ongoing.
False Positives and False Negatives
AI image detectors are not perfect. Sometimes, they mistakenly classify real images as AI-generated (false positives) or fail to detect synthetic content (false negatives). False positives can cause unnecessary concerns, while false negatives allow manipulated images to go undetected.
To reduce errors, developers train AI detection models on diverse datasets, improving their ability to distinguish between real and synthetic images. Combining multiple detection methods, such as forensic analysis, pattern recognition, and metadata inspection, can enhance overall accuracy.
Ethical Concerns and Privacy Issues
The use of AI-generated image detectors raises ethical and privacy concerns. These tools analyze image metadata, pixel structures, and compression artifacts, which may involve scanning personal photos. While the goal is to detect synthetic images, improper use of detection tools could lead to privacy violations or misuse of personal data.
Organizations must establish ethical guidelines for AI image detection. Transparency in how detection tools operate and ensuring they do not infringe on privacy rights are essential for responsible use. Regulatory bodies may need to introduce policies to govern the ethical deployment of these tools.
Industries That Benefit from AI Image Detectors
Financial Institutions and Fraud Prevention
Banks and financial institutions use AI-generated image detectors for identity verification. Fraudsters create synthetic identities using AI-generated images to bypass KYC (Know Your Customer) checks. AI detectors help banks verify whether an image is genuine, preventing identity fraud and unauthorized transactions.
Additionally, AI-generated image detection is useful in online payment security. Some attackers manipulate facial recognition systems using AI-generated photos. By identifying synthetic images, financial institutions can enhance security measures and reduce fraud risks.
Media and Journalism
News organizations and media agencies rely on AI-generated image detectors to verify images before publication. AI-generated images can be used to spread false narratives, manipulate public perception, and undermine trust in journalism. By using detection tools, media outlets can ensure that published content is authentic.
These tools also help in copyright protection. AI-generated image detection assists in tracking whether an image has been altered or used without proper attribution. This is especially important for photographers, artists, and digital content creators.
Law Enforcement and Cybersecurity
Law enforcement agencies use AI-generated image detectors in criminal investigations. AI-generated images and deepfake technology can be used to manipulate evidence, impersonate individuals, or spread harmful content. Detecting synthetic images is crucial in digital forensics and cybercrime prevention.
Cybersecurity firms also rely on AI-generated image detection to prevent phishing attacks. Attackers use AI-generated images to create fake websites, impersonate business executives, or trick users into sharing sensitive information. AI detection tools help organizations identify and block such threats.
Conclusion
AI-generated image detectors are essential in today’s digital world. As AI-generated images become more realistic, the need for accurate detection tools continues to grow. These tools help prevent fraud, combat deepfake misinformation, and maintain trust in digital interactions.
While AI detection faces challenges such as evolving AI models, false positives, and ethical concerns, continuous advancements in deep learning, forensic analysis, and blockchain verification will improve accuracy. By integrating AI-generated image detection with security systems, businesses, media platforms, and law enforcement agencies can safeguard digital content.
The future of AI image detection depends on collaboration between technology developers, regulatory bodies, and users. By staying ahead of AI-generated content techniques, organizations can ensure digital authenticity, prevent fraud, and create a more secure online environment.